In November 2017, the Singapore Economic Development Board (EDB) launched the Smart Industry Readiness Index (SIRI) and its accompanying Assessment Matrix.
In a bid to get manufacturers up to speed with industry 4.0, the three building blocks of process, technology, and organisation which SIRI lays out have been key in assessing companies industry 4.0 readiness.
The advancements of IoT have been instrumental in paving the way forward for industry 4.0, and though it is an exciting prospect for companies to jump into, and they need to understand the considerations needed to make the shift towards industry 4.0.
Firstly, they need to identify what is redundant and needs to be removed. Secondly, how to adopt existing components into the new setup. Thirdly, what is native for the new setup.
Improving the SIRI blocks of process and technology have to be balanced with practicality, depending on the current stage of a company’s technological journey, embarking on a partial or complete system overhaul would be costly, and the disruption with downtime would be impractical. Though change is necessary for our manufacturing industries to stay competitive, it does not need to be painful or drastic.
Predictive maintenance becomes the crucial factor that allows companies to fully optimise their current processes, and no matter the stage of their business. It also functions as the bridge that smoothens the transition as they build up industry 4.0 capabilities, and also a litmus test that determines the level of digitalisation that any process should adopt.
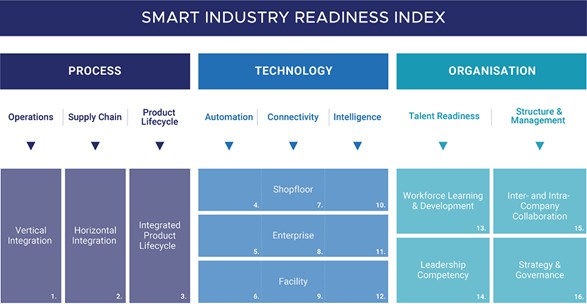
Prior to industry 4.0, maintenance in the manufacturing industry has moved through a few different phases, before landing on predictive maintenance as its latest iteration.
- Reactive maintenance - The most rudimentary approach where things are fixed when they fail, often acceptable for cheaper machines with redundant parts, this addresses the symptom and not the problem.
- Preventive maintenance - Replacing parts before they fail, this timed approach often leads to higher costs as parts are binned while having considerable mileage left, requiring more planned downtime for machines that are working perfectly well.
- Proactive maintenance - Normally reserved to reduce the cost in time and money for complex, expensive machinery, companies address the peripheral symptoms that can lead to problems and is the start to a data-driven and analytical approach.
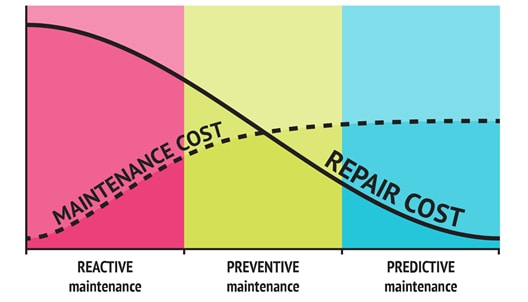
Predictive maintenance as a bridge
Though cheaper at the onset, the above-mentioned maintenance models resulted in productivity losses between 5% to 20%, which hurts any operation in the long run. By balancing costs to results, the implementation of predictive maintenance can be done progressively to serve as a bridge.
For companies that are at the starting line, examining their processes and establishing a baseline for the condition of parts and machinery involved through any historical or recorded data from their current maintenance workflow.
As the effectiveness of predictive maintenance is predicated on the quality of data, the baseline helps to identify which parts of the process the team knows most about, and which parts have the greatest room for improvement.
Moving step by step and knowing the key data points from the known allows companies to map their way to other parts of their processes that have little to no data.
Gradually giving companies a better picture to navigate their workflow to the unknown will allow companies to get a complete picture of their workflow and to ultimately decide what industry 4.0 means for them.
Predictive maintenance as a litmus test
For parts of the process that lack sufficient data to determine points of failure, businesses are often faced with a feast or famine scenario, where data is o’t collected until a failure occurs. Or sometimes the data collected is good for diagnostic purposes, but not comprehensive enough to aid in predictive maintenance. In such cases creating a visual model or simulation would have to be done first before predictive maintenance can come into play.
Predictive maintenance gives companies the flexibility of being progressive and consistent with minimal disruption, and it has to be tailored for the industry and the workflow. As detailed in a case study predictive maintenance has to be weighed carefully as an option for these reasons.
- Lack of datapoints for predictive maintenance to be truly effective
- The timelines and resources involved in the maintenance process
- Lack of significant impact, for example in the case of multiple redundancies in place
- Lack of cost savings for predictive maintenance to be financially viable
Predictive maintenance as the future
There is still plenty of room for predictive maintenance to become an industry-standard across the board. In a report done by McKinsey & Company, only 30% to 40% of production lines in ASEAN are automated.
By 2025, predictive maintenance will account for 10% to 40% reduction in spending, 3% to 5% improvement in equipment lifetime, and 50% reduction in equipment downtime. Predictive maintenance in ASEAN is projected to have an economic impact in the manufacturing industries between $38 billion to $91 billion USD by 2025.
Adopting predictive maintenance will not be an overnight process, and the Singaporean government is dedicating resources and grants to elevate the manufacturing landscapes. Companies will have to go back to the SIRI board and make sure that their people and organisations are on the same page.