In many parts of Asia, seasonal torrential rains bring with them floods that damage property and livelihood of citizens. Whereas in the past, city administrations, citizens and businesses can do almost nothing but ride out the unwelcoming waves of flood water and the potential diseases these carry, technologies like the Internet of Things (IoT), machine learning (ML) and artificial intelligence (AI) may provide respite for more forward-looking leaders.
This is the case of the DKI Jakarta Provincial Government's Flood Control System in the Jakarta Smart City application. Developed by Jakarta Smart City in collaboration with the Jakarta Water Resource Service (DSDA) to optimise flood risk management in Jakarta, the project involved the use of IoT, AI and ML as part of an early warning system against the risk of floods in the city.
As more organisations deploy IoT in commercial and industrial environments, the amount of data that is derived from these devices and sensors may prove important in improving quality, operational efficiency, and in the case of Jakarta – saving lives and property from natural disasters.
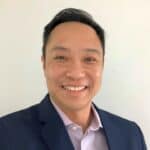
Kenneth Koh, head of industry consulting at SAS Institute, argues that the speed and accuracy with which an IoT system reacts to its environment is critical. However, with the devices and other sensors in a typical system generating overwhelming amounts of data, traditional tools and methods can slow down the process of making sense of that data.
Can you explain what AI-embedded IoT is?
Kenneth Koh: Processing data at or near the edge allows IoT systems to be nimbler and more impactful. But the quality of a data-led action is only as meaningful as the quality of the data-based insights it is acting on.
IoT by itself is not new to manufacturers. Manufacturers have been collecting and storing sensor data from machines for decades. The value proposition for them is in AIoT -analysing that data, at the edge in real-time, using AI and ML to drive efficiencies and value.
By equipping IoT systems with AI capabilities, a wide variety of data, both structured and unstructured, can be processed on the edge. High-quality insights are made available at increased speeds for systems to act on.
AI-embedded IoT and how it unlocks business value
Kenneth Koh: AI-embedded IoT improves operational efficiency and productivity while reducing costs. It also drives innovation towards better customer service, better products, and faster product deployment to the market.
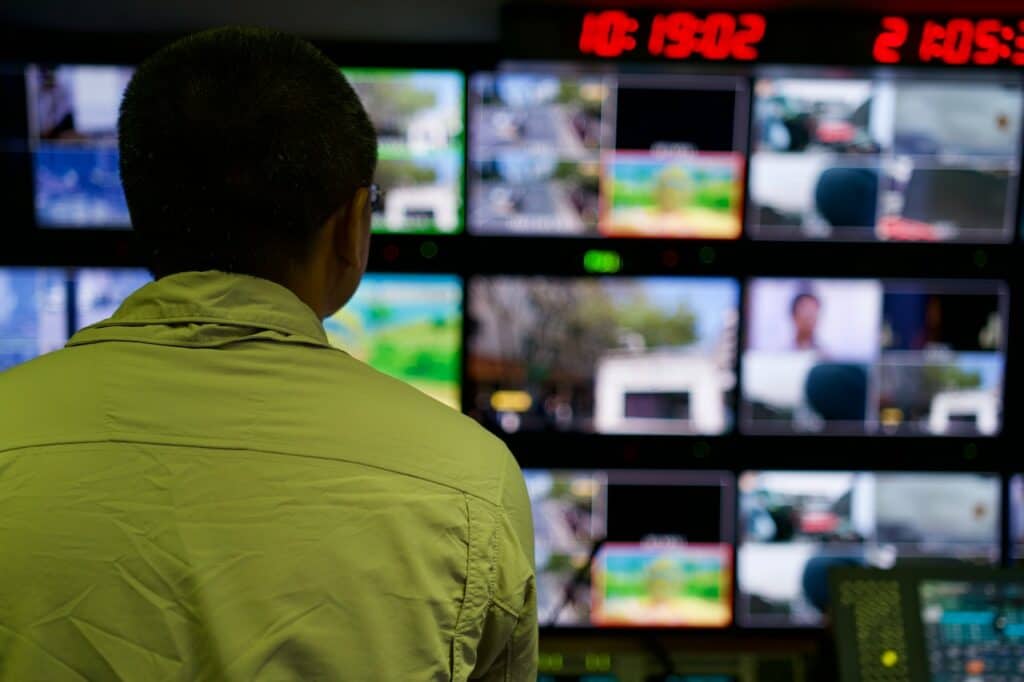
Embedding AI in IoT devices enables edge computing, allowing for the deployment of IoT systems in situations where consistent 5G networks are unavailable. For example, logistics providers can use IoT sensors in their transportation fleet to monitor the internal and external conditions in their vehicles, even in remote parts of the latter's routes.
Aside from edge computing, AI-embedded IoT utilises machine learning to develop actionable insights from the terabytes of data an IoT system generates daily. In the example above, data collected from these sensors are sent to the cloud in real-time, enabling technicians to address vehicle breakdowns more accurately and much faster.
Manufacturers can also use these insights to predict when a particular factory system or piece of equipment would fail, allowing technicians to implement preventive maintenance. Proactive detection of faulty equipment saves precious man-hours while reducing costly unscheduled downtimes.
On the retail side, insights from IoT systems can be used to identify optimal prices for products and minimise disruption to their supply chains.
ML and its role in IoT analytics
Kenneth Koh: Machine learning is the edge AI-embedded IoT has over other IoT deployments. Systems can learn as it processes sensor-generated data using diverse advanced analytical methods such as decision trees, random forests, gradient boosting, neural networks, support vector machines, and factorization machines.
This creates savings for enterprises in terms of man-hours and specialists in the organisation. Without the need to extensively train AI systems, specialists can focus on other critical tasks as non-data scientists can access, view, and process data.
Machine learning capabilities also increase the range of data that AI systems can access and process: visual images online and offline, text, and even verbal speech. The increased volume and quality of available data increases the value and impact of insights derived from it.
Combined, these machine learning capabilities enable both increased speed and volume of data processing, enabling real-time actionable insights which are crucial in many IoT systems.
How AIoT supported Jakarta Smart City: Using SAS's AI-powered platforms, Jakarta Smart City was able to integrate multi-source data in real-time and provides advanced analytics with IoT, machine learning & AI technology to provide emergency/disaster predictive capability and optimisation to serve the public. The result is a flood emergency response to mitigate the risk of floods in Jakarta.
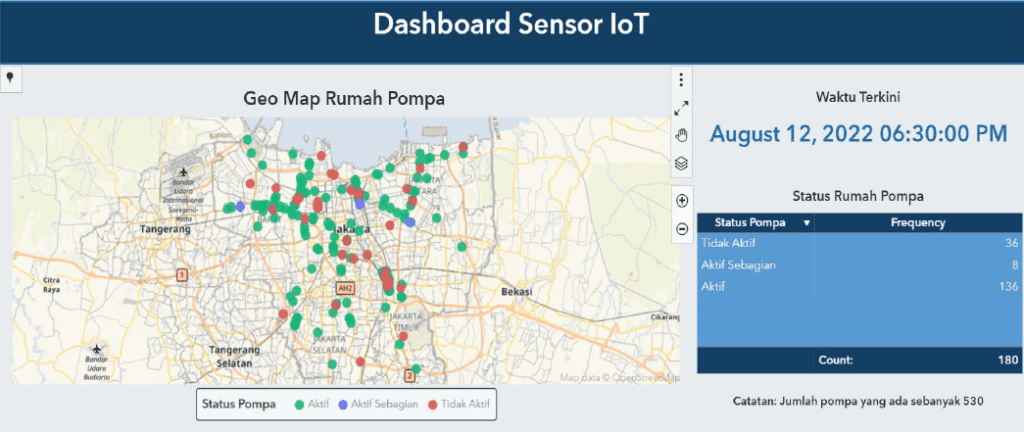
Source: SAS, Priority Consultants
Given that IoT historically falls under operational technology, who should own the securing of IoT?
Kenneth Koh: The introduction of IoT is blurring the lines between IT and OT across enterprises. Sensors and devices are connected to the network to create new systems and improve processes. At the same time, this convergence exposes traditional OT equipment and systems to threats they were previously insulated from.
The fact is that true device security is a combination of technologies, processes, and best practices. Thus, securing IoT systems should not be the sole domain of either OT or IT teams but engendering closer, more effective collaboration between both.
However, this is easier said than done, as IT security teams and OT security teams often don't speak the same language and find it difficult to understand each other's point of view.
Responsibilities are distributed quite differently; priorities often diverge and regulations governing OT security and IT security can sometimes contradict one another. Gaining an overview of all assets within the given environment makes clear what assets and processes must not fail under any circumstances.
By doing this, organisations can establish and practice unified cybersecurity that ensures the confidentiality, integrity, and availability of data.
Name one best practice for IT and operational technology staff working together.
Kenneth Koh: In manufacturing, data is very time sensitive. For example, if the chemical concentration of a process is drifting away from optimal, the engineer may have only minutes to react to save many tons of product.
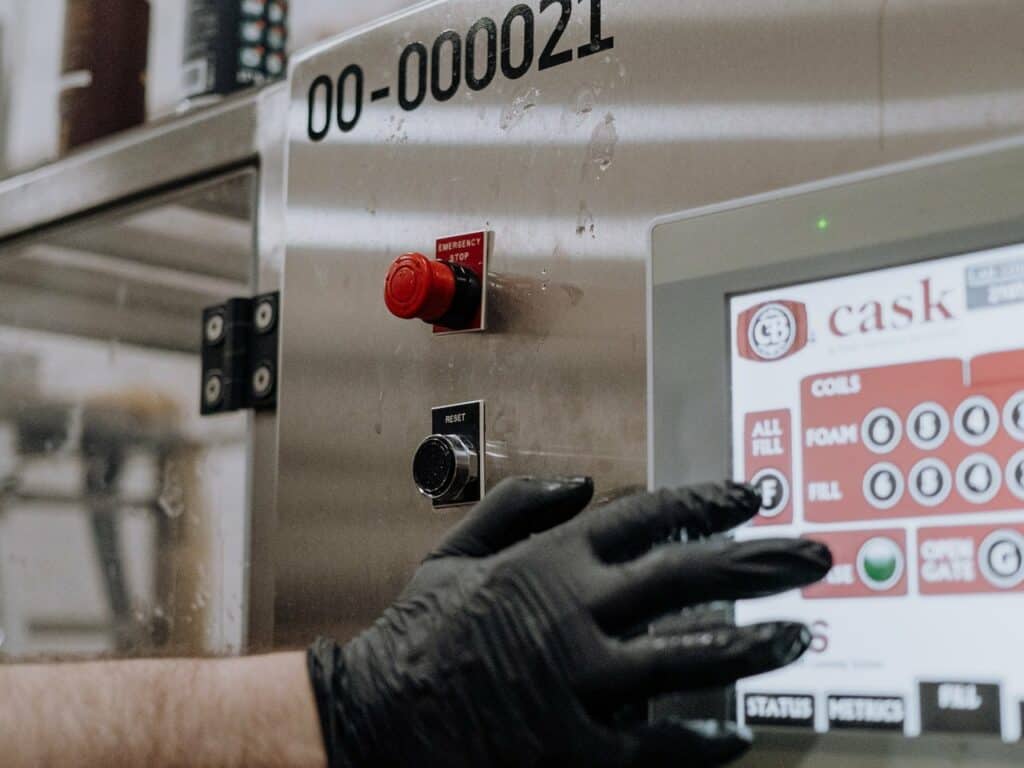
In many semiconductor processes, engineers have only seconds to react. In such situations, Analytics needs to move to the ‘edge’ which means the data must be analysed and decisioned at the machine or on the shop floor, not in the back office or engineering.
This requires the ability to do analytics wherever it is needed –at the machine, on the production floor, in the cloud or the back office.
One of the major challenges faced is data silos. For organisations that are not implementing IT/OT convergence, due to a patchwork of unintegrated or partially integrated apps and enterprise systems. Without careful planning, introducing new sources of data (e.g. IoT sensors) will compound the problem.
Implementing a data integration platform to connect IoT systems with organisations' existing technology stack breaks down silos between historical and future data while providing all teams the same access through a single point of control. This ensures that IT and OT teams are with the same page, establishing a foundation for better IT/OT convergence.